As a follow-up to a previous post about AI in education, we wanted to take a closer look at how the bias baked into artificial intelligence can affect learners. We wondered how bias shows up in the field of education and how the use of artificial intelligence results in prejudice and changes the landscape for already vulnerable learners. Through examples from the field, we found that there is much work to be done.
Bias in Education
Our classrooms are already rife with unconscious bias. This bias exists because schools are first and foremost human places, and we humans bring bias with us wherever we go. As teachers, we bring our socio-cultural baggage into the classroom, where it co-exists with the socio-cultural baggage of our students.
At best, this baggage is responsible for us liking some students more than others. At worst, our baggage impacts our teaching and evaluation practices and therefore the success of our students. Student success, or lack of it, affects their choices long after they leave our classroom.
Consider a study conducted in 2015 at the University of Tel Aviv. This study involved teachers scoring student math tests. Sounds straightforward, right? Math is either correct or incorrect, so bias should absolutely not affect test scores. Conventional wisdom suggests that Math and especially math testing is one of those places where bias should not operate.
The research was carried out on three groups of students in Israel from sixth grade through the end of high school. The students were given two exams, the first graded by objective scorers who did not know their names and the second by instructors who did know them. In math, the girls outscored the boys in the test that was scored anonymously, but when graded by teachers who were familiar with their names, the boys outscored the girls. The effect was not the same for tests in non-math or science-related subjects.
The researchers concluded that, in math and science, the teachers overestimated the boys’ skills and underestimated the girls’ abilities, and that this had long-term implications for students’ attitudes toward these subjects. (emphasis added)
The Tel Aviv University 2015 study focused on gender. Other studies on bias in education focus on racialized communities or Indigenous students. Often due to the homogeneity of school staff – in Canada, overwhelmingly white and female – school-based bias affects student attendance, attitudes towards school, academic outcomes and completion rates. Homogeneity – when a group of people share similar demographic characteristics – is the perfect incubator of unconscious bias. This is mainly due to not having anyone to challenge preconceived notions or provide different perspectives and nuances. A homogenous group will share a similar worldview and will have nobody within it to provide alternate ways of knowing and being. This is true no matter how perceptive or self-aware specific individuals in that group happen to be. TL;DR – bias is inevitable.
AI Bias in Education
Why are we discussing the bias that exists in our schools when this blog post is supposed to be about bias in AI? Artificial intelligence, the kind that is built into software and apps, was built by humans. A very specific group of humans, actually – computer scientists. Joy Buolamwini of the Algorithmic Justice League points out what she calls the “pale male data problem” in her Time magazine article:
Less than 2% of employees in technical roles at Facebook and Google are black. At eight large tech companies evaluated by Bloomberg, only around a fifth of the technical workforce at each are women. I found one government dataset of faces collected for testing that contained 75% men and 80% lighter-skinned individuals and less than 5% women of color—echoing the pale male data problem that excludes so much of society in the data that fuels AI. (Buolamwini, 2019)
What does this mean for education?
In addition to programmer biases that may be present in AI algorithms, bias can also be found in the data sets that AI software pulls from to generate new content. Even when data sets are large, they are products of the humans that produce them. This means dominant societies’ beliefs, values, and histories also tend to dominate AI output, thereby perpetuating issues related to diversity, equity and inclusion already present in society and schools.
How does this play out in the student experience?
Let’s take a look at the popular DALL-E 2, an AI system that can create realistic images and art from a description in natural language. When given the prompt “a cartoon girl riding a unicorn over a rainbow,” – DALL-E 2 offered up exclusively figures with Eurocentric features like pale skin. For students, lack of representation and the undue valuing of specific features creates erasure and the feeling of not seeing oneself in the learning environment.
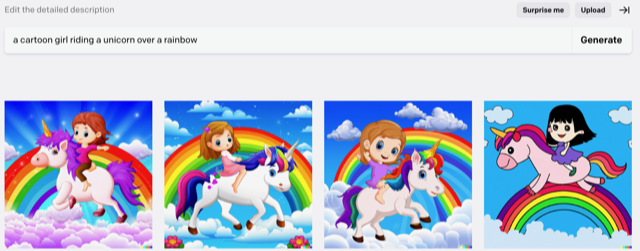
Dall E 2 Generated photos using the prompt “a cartoon girl riding a unicorn over a rainbow”
Other text-to-image generators are:
depicting certain care professions (dental assistant, event planner) as nearly exclusively feminine, or positions of authority (director, CEO) as exclusively masculine. . .. These biases can then participate in the devaluation of certain kinds of work or putting up additional barriers to access to careers for already under-represented groups. (Luccioni and Akiki, et al., 2003)
This means that student perceptions about different jobs and the career barriers they might face can be impacted by text-to-image generators, thus influencing their career choices and educational decisions. While schools at all levels of learning have been working to highlight diversity in different professions, some text-to-image generators are a step in the opposite direction.
Another example can be found in language-learning software:
Language-learning software that takes into account how students speak, but is often trained on data from native-English speakers born in America and treats other accents and dialects as wrong. (Herald, 2022)
Learners speaking a different form of English – like African American Vernacular English, or the English spoken by Indigenous people in Canada, or even that spoken by people with an accent – would be at a disadvantage when using AI-driven language-learning software.
Next steps
As educators, we are asked to mobilize digital technologies while acting as cultural facilitators.
These are two of the Professional Competencies.
To do this effectively, we need to activate our and our students’ digital competency—particularly Dimension 11, which relates to critical thinking about and evaluating technology. Classroom activities and discussions on how AI and other technologies have and will continue to affect daily life and societal beliefs should become increasingly prevalent. Such activities and discussions can help students and educators decide what technological tools support their school goals and positively contribute to their school climate. If you are looking for digital competency information, lesson plans and other supports, we encourage you to check out the resources section of the Digitial Competency in Action website.
AI technology is rapidly advancing, and there are a lot of new technologies for educators (and students) to be aware of. One of the best ways to stay updated with the newest technologies is to follow online resources. There are lots of news sources and blog sites that cover emerging AI technologies. Companies that are developing AI tools, like OpenAI, Apple, Google, and Microsoft, also have a section on their websites where you can read updates about their current products and research. Finding time to connect with colleagues about new AI software and technologies can also be helpful. As a staff, you could research and try out different AI tools and discuss if and how you might use them with students.
Many schools have committed to teaching and advocating for equity, diversity and inclusion. They want to welcome students from diverse backgrounds and create spaces where students see themselves reflected in their academic environments. As schools use more AI technology, we must be aware that some of these tools can amplify existing societal biases and offset the strides we have already made toward promoting multiculturalism. In this way, nurturing digital competency is essential to our commitment to inclusive schools. Not all bias is necessarily bad, but we should strive to become aware of our prejudices and biases in technology because they can distort the truth. Awareness of bias can help mitigate potential negative impacts. To date, AI software does not assess the reliability or accuracy of content in the same way a human would. Nor does AI software reflect on how the content it generates impacts human societies. While the latest AI tools can do pretty neat things, school boards, students and teachers still need digital competency to evaluate whether these tools positively contribute to their visions for an inclusive society.
Want to read more?
Here is a Datacamp post which provides a few clear examples of three common types of AI bias. aiEDU has also developed some challenges that could be explored by teachers and students looking to learn more about AI and how people are working to build and use AI ethically.
Some AI companies are trying to develop software to help reduce bias and promote diversity, equity and inclusion. For example, Mila, the Quebec Artificial Intelligence Institute, is working on an AI project called Biasly to help detect and correct misogynistic language. This project is projected to be released in the fall of this year.
The Center for Humane Technology has podcasts and resources to explore the responsible use of technology. The March 9th, 2023 Podcast by Tristan Harris and Aza Raskin, titled The A.I. Dilemma, contains commentary about the deployment of new AI technologies and how they are and will continue to impact society.
Duolingo Max is already beginning to add simulated AI conversations to its language-learning software, highlighting some of the ways AI-generated content might support student learning. FranklyAI’s Te Reo Māori chatbot out of Australia and New Zealand is also working to promote the revitalization of non-dominant languages and customs. This AI chatbot centers around Māori customs and allows users to interact using multiple Māori dialects. This makes us think about how AI could potentially support revitalizing Indigenous languages currently spoken in Canada by providing opportunities to practice languages in simulated conversations.
Bibliography
Buolamwini, Joy (2019). Artificial Intelligence Has a Problem With Gender and Racial Bias. Here’s How to Solve It. Time Magazine, Feb 7, 2019. https://time.com/5520558/artificial-intelligence-racial-gender-bias/
Coded Bias – Documentary
https://www.codedbias.com/
Herold, Benjamin. (2022). Why Schools Need to Talk About Racial Bias in AI-Powered Technologies. https://www.edweek.org/leadership/why-schools-need-to-talk-about-racial-bias-in-ai-powered-technologies/2022/04 , accessed April 17, 2023
Racism contributes to poor attendance of Indigenous students in Alberta schools: New study
https://theconversation.com/racism-contributes-to-poor-attendance-of-indigenous-students-in-alberta-schools-new-study-141922 , accessed April 17, 2023
Luccioni and Akiki, et al. (2023) Stable Bias: Analyzing Societal Representations in Diffusion Models. https://arxiv.org/pdf/2303.11408.pdf, accessed April 19, 2023
World Economic Forum Artificial Intelligence for Children Toolkit. (March 2022) https://www3.weforum.org/docs/WEF_Artificial_Intelligence_for_Children_2022.pdf, accessed April 17, 2023
Photo credits
A Cognitive Bias Codex. Showing 180+ biases. designed by John Manoogian III from Wikimedia Commons
Unconscious bias sketch notes by Claudio Nickele based on a talk given by Brigitte Lipschutz from a Flickr photo
Photos generated by DALL.E 2 on April 21, 2023 with the prompt: A cartoon girl riding a unicorn over a rainbow
Scrabble word photo by wiredsmartio on Pixabay